Elevate Your Data Science Expertise with Best Course
- fewono5705
- Jun 5, 2024
- 6 min read
From healthcare to finance, retail to technology, data science is transforming industries by turning vast amounts of data into actionable insights. However, to harness the power of data science effectively, quality education and training are paramount. This article will guide you through the essentials of selecting the best data science course to elevate your expertise and advance your career.
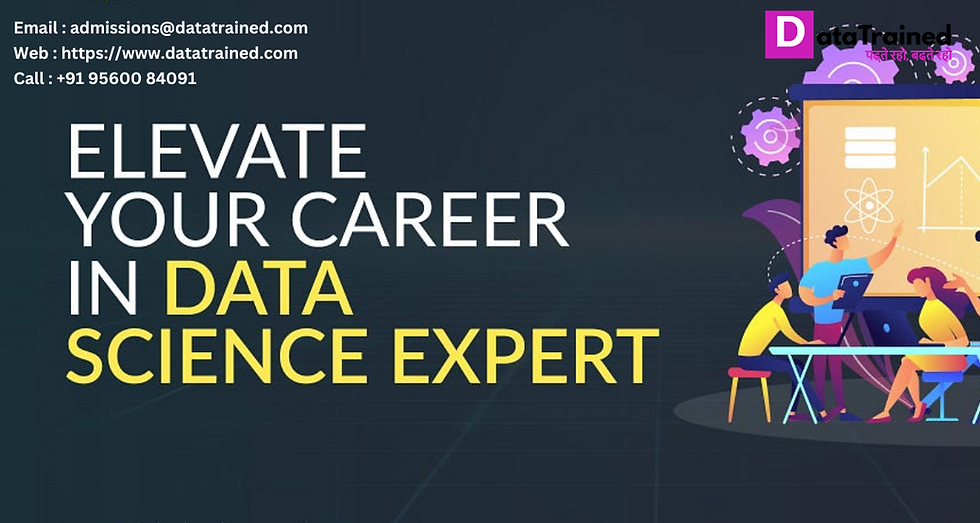
What to Look for in a Data Science Course
Accreditation and Certification
When choosing a data science course, the first thing to consider is accreditation. Accredited courses ensure that the curriculum meets industry standards and that the certification you receive upon completion is recognized by employers. Also read: Enroll in Data Science Course with Placement Guarantee.
Curriculum Coverage
A comprehensive curriculum that covers a wide range of topics—from statistics and machine learning to data visualization and big data technologies—is crucial. Look for courses that offer a balanced mix of these subjects.
Practical vs. Theoretical Balance
Data science is an applied field, so a good course should strike a balance between theory and practical application. Hands-on projects, case studies, and real-world datasets are essential components of an effective data science education.
Instructor Expertise
The instructors’ qualifications and experience play a significant role in the quality of the course. Look for courses taught by industry professionals or academics with substantial experience in data science. Also read: Get started with Data Science Classes near you.
Reviews and Testimonials
Reading reviews and testimonials from previous students can provide insights into the course's effectiveness, teaching style, and overall value. This feedback is invaluable when making your decision.
Top Data Science Courses Available
Coursera's Data Science Specialization by Johns Hopkins University
Overview
This specialization covers the entire data science process, from data collection to publication. It consists of ten courses, culminating in a capstone project.
Key Features
Comprehensive coverage of data science topics
Taught by experienced faculty from Johns Hopkins University
Flexible schedule
Pros and Cons
Pros:
Highly regarded certification
Strong focus on practical skills
Cons:
Requires a significant time commitment
Data Science MicroMasters Program by edX (MIT)
Overview
MIT's MicroMasters program offers a series of graduate-level courses designed to provide deep knowledge in data science. Also read: Start your Data Scientist Classes to enhance your skill-sets.
Key Features
Rigorous academic curriculum
Hands-on projects and assignments
Option to credit towards a full Master's degree
Pros and Cons
Pros:
Prestigious certification
In-depth theoretical and practical knowledge
Cons:
Higher cost compared to other courses
Udacity's Data Scientist Nanodegree
Overview
Udacity's Nanodegree program is tailored to equip students with job-ready skills in data science.
Key Features
Real-world projects designed by industry experts
Mentorship and career support
Pros and Cons
Pros:
Strong focus on practical applications
Excellent career services
Cons:
Less focus on theoretical concepts
Harvard's Data Science Professional Certificate
Overview
Offered through edX, this program provides a solid foundation in data science, emphasizing critical skills like R programming, statistical inference, and machine learning. Also read: Learn the Data Science Full Course from DataTrained Today!
Key Features
Taught by Harvard faculty
Hands-on projects using real-world data
Pros and Cons
Pros:
Prestigious institution
Balanced curriculum
Cons:
May require prior knowledge in statistics
Simplilearn's Data Science Certification Training
Overview
Simplilearn offers a comprehensive data science certification that includes live online classes, self-paced videos, and projects.
Key Features
Extensive course material
Industry-recognized certification
Pros and Cons
Pros:
Flexible learning options
Practical focus
Cons:
Varying instructor quality
Why Coursera’s Data Science Specialization Stands Out
Comprehensive Curriculum
Coursera's specialization by Johns Hopkins offers a broad and deep curriculum that covers all essential areas of data science. This ensures you gain a well-rounded education.
Industry-Relevant Projects
The course includes projects that mirror real-world data science challenges, providing practical experience that is directly applicable to the workplace. Also read: Get your IBM Certified Data Science Degree along with Certificate Today!
Flexibility and Accessibility
With flexible scheduling and online access, you can learn at your own pace, making it ideal for working professionals.
Benefits of Completing a Data Science Course
Career Advancement
A data science certification can significantly boost your career prospects, opening doors to roles such as data analyst, data scientist, and machine learning engineer.
Skill Enhancement
You’ll acquire both technical and analytical skills that are highly sought after in the job market.
Networking Opportunities
Courses often provide access to a community of peers and professionals, which can be invaluable for networking and career growth.
Higher Earning Potential
Data scientists are among the highest-paid professionals in the tech industry, and completing a reputable course can increase your earning potential.
How to Choose the Right Course for You
Assessing Your Current Skill Level
Evaluate your existing knowledge and skills to choose a course that matches your level—whether beginner, intermediate, or advanced.
Identifying Your Career Goals
Consider what you aim to achieve in your career. Different courses may cater to various aspects of data science, from analytics to machine learning.
Considering Your Learning Style
Some people thrive in structured environments, while others prefer self-paced learning. Choose a course format that aligns with your learning preferences.
Budget and Time Constraints
Consider how much time and money you can invest. Some courses are free or low-cost, while others require a significant investment.
Preparing for a Data Science Course
Prerequisites You Should Know
Familiarize yourself with the basic prerequisites, such as programming (Python/R), statistics, and linear algebra. Many courses provide preparatory material.
Setting Up Your Learning Environment
Create a conducive learning environment with the necessary tools, software, and resources. This might include setting up a dedicated workspace and ensuring reliable internet access.
Tools and Software to Familiarize With
Learn about the tools and software commonly used in data science, such as Jupyter Notebooks, RStudio, and various libraries in Python (e.g., pandas, scikit-learn).
Maximizing Your Learning Experience
Active Participation in Forums and Discussions
Engage actively in course forums and discussion groups. Asking questions and sharing knowledge can enhance your understanding and retention.
Regular Practice and Hands-On Projects
Consistent practice and completing hands-on projects are crucial. They reinforce theoretical knowledge and develop practical skills.
Seeking Feedback and Continuous Improvement
Seek feedback on your projects and assignments to identify areas for improvement. Continuously refine your skills based on this feedback.
Applying Your Knowledge in Real-World Scenarios
Internship and Job Opportunities
Look for internships and entry-level job opportunities to apply what you've learned. Practical experience is invaluable.
Freelance Projects
Consider taking on freelance projects. Platforms like Upwork and Freelancer can help you find data science projects to build your portfolio.
Building a Portfolio
Create a portfolio showcasing your projects, skills, and achievements. A well-organized portfolio can make a significant difference in job applications.
Networking and Community Engagement
Joining Data Science Communities
Participate in online communities such as Kaggle, GitHub, and LinkedIn groups. These platforms offer opportunities for collaboration and learning.
Attending Conferences and Meetups
Attend data science conferences, webinars, and meetups to connect with professionals and stay updated on industry trends.
Online and Offline Networking Strategies
Leverage both online platforms and offline events for networking. Building relationships in the data science community can lead to mentorship and job opportunities.
Staying Updated in the Field of Data Science
Continuous Learning and Upskilling
The field of data science is rapidly evolving. Engage in continuous learning by taking advanced courses and certifications.
Following Industry Trends and News
Stay informed about the latest trends, technologies, and research in data science by following reputable blogs, journals, and news outlets.
Enrolling in Advanced Courses
As you progress, consider enrolling in advanced courses to deepen your expertise and stay competitive in the job market.
Common Challenges and How to Overcome Them
Time Management
Balancing learning with other responsibilities can be challenging. Create a schedule and set realistic goals to manage your time effectively.
Keeping Up with Rapid Changes
The rapid pace of change in data science can be daunting. Focus on mastering foundational concepts and continuously update your knowledge.
Balancing Theory and Practice
Ensure you’re applying theoretical knowledge through practical projects. This balance is crucial for becoming a proficient data scientist.
Success Stories: Inspiring Journeys in Data Science
Profiles of Successful Data Scientists
Learn from the experiences of successful data scientists. Their journeys can provide valuable insights and inspiration.
Key Takeaways from Their Experiences
Understand the key strategies and decisions that contributed to their success, such as continuous learning, networking, and hands-on practice.
In Conclusion, Elevating your data science expertise through a quality course is a strategic investment in your future. By carefully selecting the right course, actively engaging in the learning process, and applying your knowledge in real-world scenarios, you can unlock new career opportunities and achieve significant professional growth.
Opmerkingen